Hypothesis generation for drug discovery
Features of Drug Discovery AI Factory
Novelty
Discovery of unreported target genes with high disease relevance utilizing self-developed AI “KIBIT”
High accuracy
Unique analysis methods extract target genes with high probability of success
Hypothesis generation
Create gene network and generate hypothesis for unreported target genes
Extraction of many unreported target genes by utilizing KIBIT
Narrowing down the target genes to even more precise targets utilizing original analysis methods
KIBIT generates networks that maps the connections between genes associated with a disease. In this process, KIBIT also predicts target genes even if their relationships to the disease are not described in the paper.
To extract targets with a high probability of success from these target genes, we utilize our own innovative analysis methods, the Drug Discovery Best Known Method (DD-BKM), to narrow down the targets with high potential for new drugs.
Example: Gene network for disease A
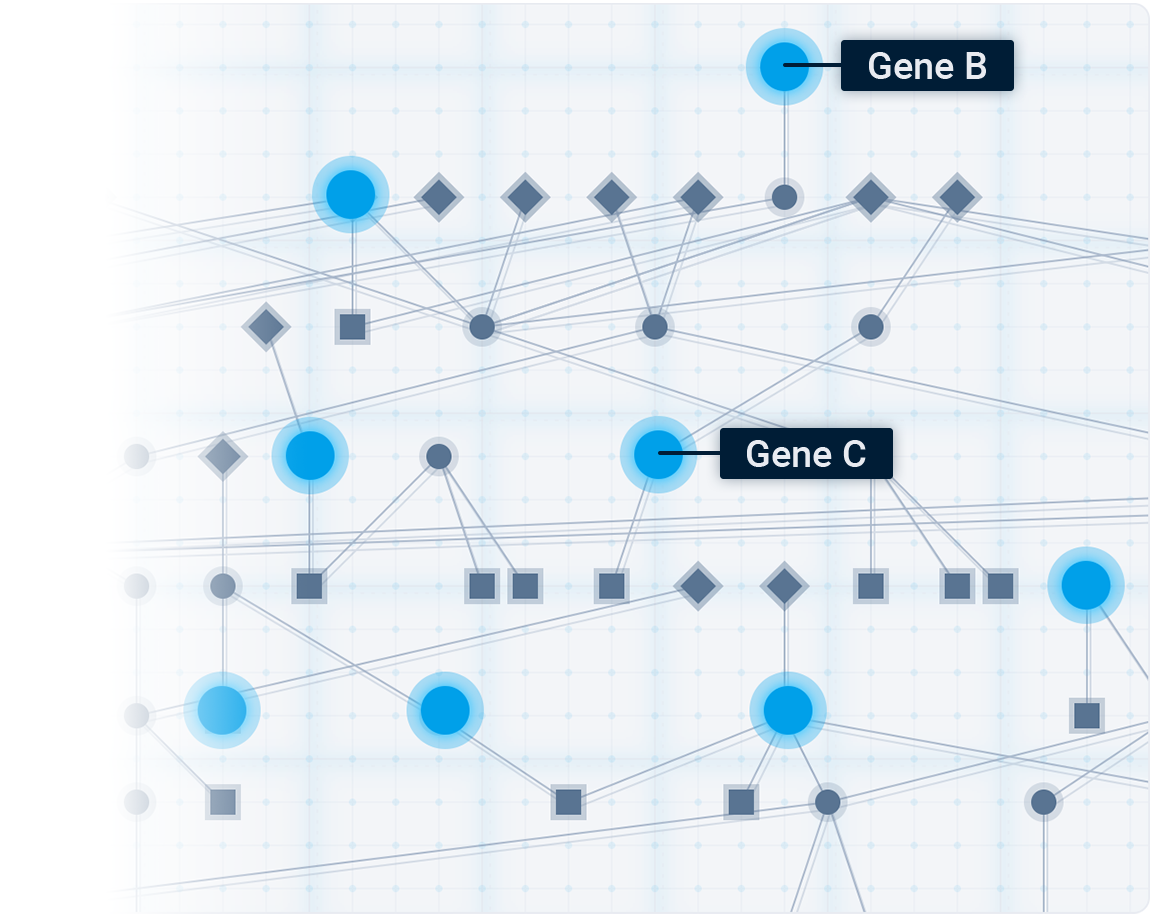
No linkage to disease A has been reported for both gene B and gene C
Extraction of genes with high probability of drug discovery success
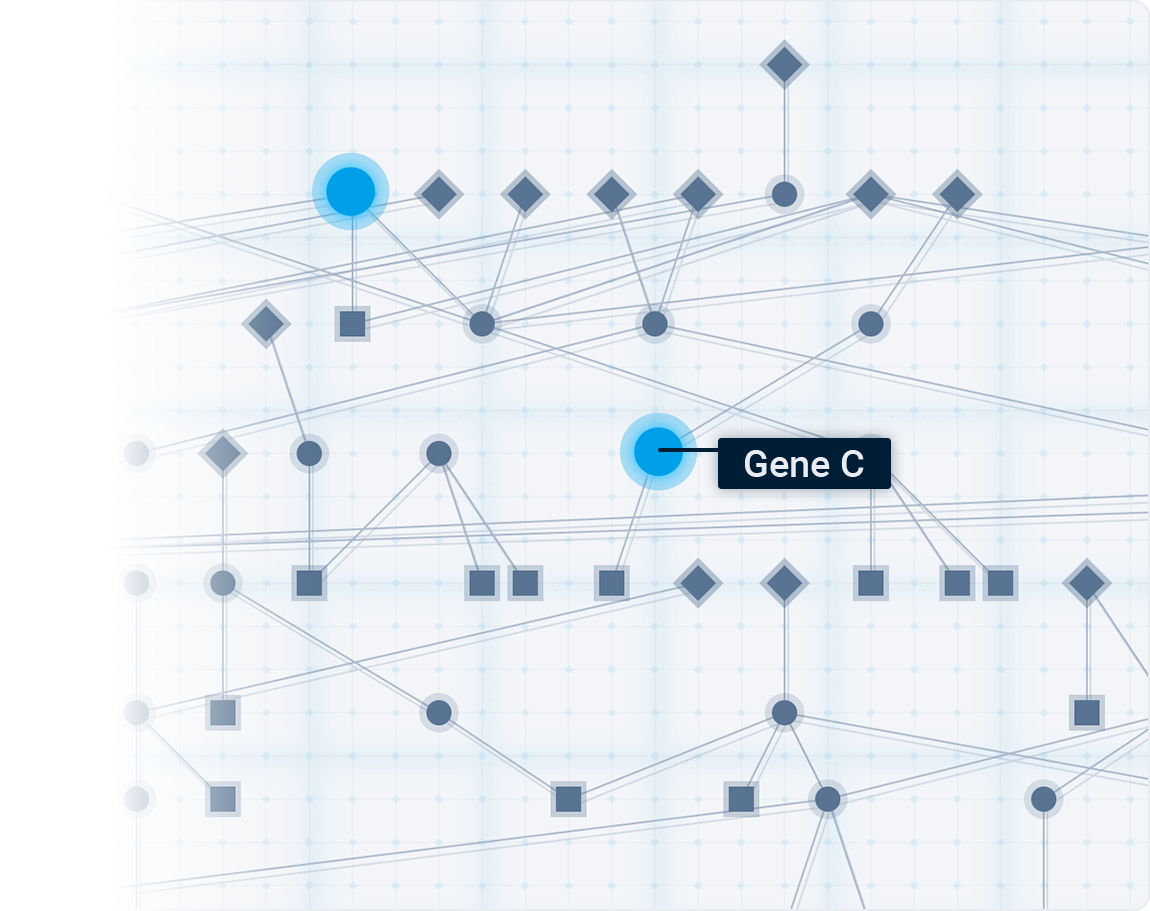
Analysis example
Virtual Experiments with KIBIT
We simulate how pathways are changed by hypothetically knocking out (KO) the target gene.
For example, in the figure below, a hypothetical KO of gene B has almost no effect on the network as it is only replaced by gene D. On the other hand, when gene C is knocked out, the network changes significantly, suggesting that gene C is the gene affecting the disease.
Unique technology to discover unknowns from known information
Predicts even genes that have no association with disease in papers
The main source of article data for analysis is PubMed, and Springer Nature article data can be added as an option. FRONTEO's drug discovery support platform is characterized by its ability to extract novel target genes from previously reported information. Genome information and OMICS data can also be added to the analysis.
KIBIT can read known article information and derive new relationships.
Analysis example
Springer Nature
Extended function
Analyzes full-text data of about 600 journals published by Springer Nature
Promoting innovative discoveries based on a variety of cross-disciplinary findings by analyzing data from biomedical, biotechnology, and other fields
Comparison of full text analysis and abstract analysis
Increasing the number of genes analyzed by KIBIT allows for the discovery of more novel target genes.
Bridges the time lag between being reported in abstract and discovering target genes on average 5 years earlier
Experts with years of experience in drug discovery research make proposals that meet customer needs
To analyze highly specialized information and derive innovative outputs, drug discovery researchers with expertise in both drug discovery and AI is essential. FRONTEO brings together a team of researchers who have long been engaged in drug discovery at pharmaceutical companies and research institutions to realize useful proposals, including appropriate target genes and indications and their hypotheses, in line with the needs of our clients.
FRONTEO's idea of hypothesis generation
The hypothesis to initiate the development of a new drug includes
- Target molecule: A gene or molecule etc., that is predicted to be associated with a disease.
- Disease mechanism: How the target molecule is related to the disease, etc.
- Patient information: Genome information, diseases, symptoms, etc., of the target patient group.
- Safety information: Toxicity of the drug candidate, etc.
- Feasibility: Proposed experimental model, etc.
FRONTEO believes that all of these factors need to be considered.