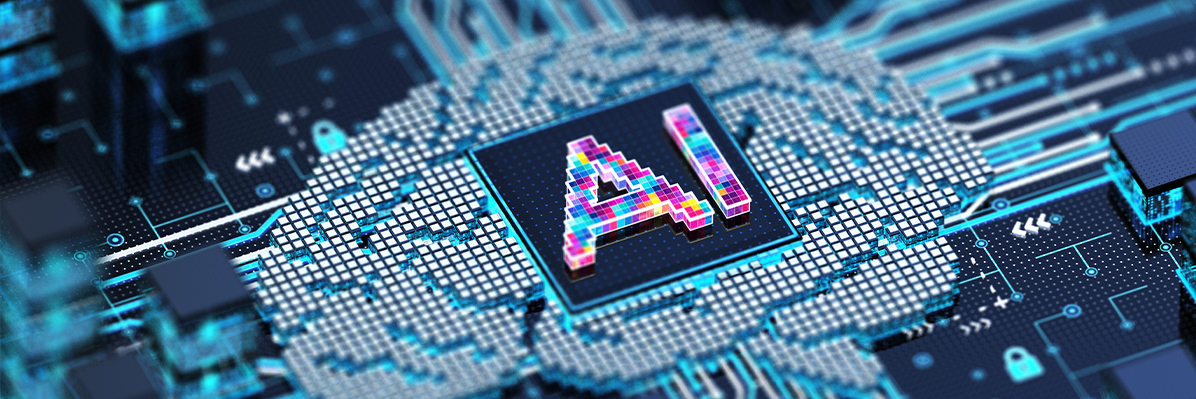
Credit: BlackJack3d/GettyImages
The following is a reprint by our company of the article advertisement, "AI can hunt for hidden clues to new drugs in published papers," originally published in the international multidisciplinary science journal Nature. The content on this page is posted under the responsibility of FRONTEO, Inc..
Our AI-driven drug discovery support service, Drug Discovery AI Factory, collaborates with Springer Nature to analyze research articles published in their specialized journals. This article highlights the unique AI technology utilized by Drug Discovery AI Factory, which uncovers unknown insights from known information. We invite you to take a look.
AI can hunt for hidden clues to new drugs in published papers
Adding context to natural language programming helps unearth unexpected connections buried in existing drug discovery literature.
Artificial intelligence (AI) offers the tantalizing promise of revealing new drugs by unveiling patterns lurking in the existing research literature. But efforts to unleash AI’s potential in this area are being hindered by inherent biases in the publications used for training AI models.
By adopting an approach that mimics the strategies children use to understand unfamiliar words when encountering1, a Japanese company is seeking to bypass this limitation. FRONTEO Inc., an AI-solutions company, with its headquarters in Tokyo, has developed a natural language processing (NLP) model that adds a critical parameter — context — to the AI-powered analysis of research literature.
“When children encounter an unfamiliar word, they grasp its meaning by looking at the surrounding context,” says Hiroyoshi Toyoshiba, chief technology officer at FRONTEO. “Similarly, our engine automatically determines meanings based on context, without relying on pre-existing definitions.”
Promising results obtained by applying this approach hint that it could lead to ground-breaking health discoveries.
Context is king
FRONTEO’s flagship AI engine, KIBIT, uses the distributional hypothesis to analyse word relationships in written texts. Formalized in the 1950s, the distributional hypothesis states that words derive their significance from their context. For example, “king” and “monarch” both appear in sentences about ruling, whereas seeing “bank” in sentences about financial institutions and rivers reveals some words have multiple interpretations.
“KIBIT focuses on the ‘company’ a word keeps, the surrounding words and their distribution,” says Toyoshiba. “This allows us to identify true connections.”
Refined over nearly two decades, the KIBIT engine excels at discovering relevant information from large datasets, such as legal documents, medical records and financial data. By creating vector representations of words based on their contexts, KIBIT uses a mapping approach to visualize data relationships, helping generate innovative hypotheses and insights.
Toyoshiba is leading the initiative to explore KIBIT’s use in drug discovery. A mathematician with expertise in computational biology and AI, he became interested in NLP while working at a pharmaceutical company. It was there that Toyoshiba recognized NLP’s potential to streamline the processing of vast amounts of scientific literature.
“When I joined FRONTEO in 2017, we improved KIBIT further to create an algorithm that analyses entire sentences and words simultaneously, making document comparisons more efficient,” recalls Toyoshiba. “Unlike most AI systems that need expensive hardware, our method works on standard computers, making it more accessible and cost effective.”
More genes, more answers
Most NLP-based approaches to literature analysis follow direct, sequential links between entities. For instance, these methods might connect findings such as “protein X interacts with protein Y” and “protein Y is involved in cellular process Z” to posit that “protein X may influence process Z”. This approach is similar to the one that researchers typically employ when reading a paper. It is difficult to uncover a completely new association using this approach, since other researchers can also derive results in the same way.
KIBIT harnesses ‘non-continuous discovery’ to extract deeper meaning from scientific literature. As an example, Toyoshiba points to queries that used PubMed or KIBIT to find genes related to amyotrophic lateral sclerosis (ALS), a progressive neurodegenerative condition that usually kills sufferers within two to five years.
PubMed’s best match identified 13 genes, mostly well-known ones with numerous publications. In contrast, KIBIT’s engine flagged 44 genes, including many less-studied ones. By examining both direct and indirect connections, KIBIT minimizes bias towards the most popular genes.
For example, KIBIT identified a specific genetic change, known as a repeat variance, in the RGS14 gene in 47% of familial ALS cases. This finding is significant because identifying this genetic change in a hereditary form of the disease could help researchers understand its causes.
The potential savings of this approach are significant as it typically costs pharmaceutical companies millions of dollars, and takes several years, to discover and validate target genes.
▶ The usually fatal neurodegenerative disease amyotrophic lateral sclerosis (ALS) causes degradation of motor neurons. Using KIBIT, researchers at FRONTEO have identified 44 genes — far more than previous studies — that may be related to ALS. Credit: KATERYNA KON/Science Photo Library/GettyImages
Sparking creativity
Another tool in FRONTEO’s drug-discovery programme, the KIBIT Cascade Eye, is based on spreading activation theory. This theory from cognitive psychology describes how the brain organizes linguistic information by connecting related concepts in a web of interconnected nodes. When one concept is activated, it triggers related concepts, spreading like ripples in a pond.
KIBIT Cascade Eye represents concepts as vectors in a multidimensional space and connects them based on a measure of how closely related they are. This could help to visually identify complex molecular interactions by revealing connections that are not immediately obvious without this arrangement.
By employing this approach, it should be possible to identify new research targets. “KIBIT Cascade Eye combines all kinds of molecular relationships to stimulate target discoveries,” explains Toyoshiba. “By mapping these connections, we can create a comprehensive network that highlights potential areas of research.”
Traditional approaches for analysing literature on diseases such as PubMed searches often prioritize well-documented genes, since they rely on publication frequency to identify established connections, Toyoshiba notes.
Overlooked genes
For instance, genes such as CYP2E1, CYP3A4 and ABCB11 frequently appear in the literature in relation to drug-induced liver injury, reflecting well-known associations commonly investigated in liver toxicity and drug-safety studies.
In contrast, KIBIT Cascade Eye excels in uncovering hidden relationships. It identified genes with few or no PubMed hits, such as MAT2A, ADH4 and ZFYVE19, but with significant AI-calculated spreading activation scores, suggesting their potential relevance.
“For example, ZFYVE19 doesn’t appear in any liver-related publications, but KIBIT Cascade Eye connects it to HNF4A, which inhibits hepcidin, causing ferroptosis and affecting liver cells,” says Toyoshiba. “Even without direct publications linking ZFYVE19 to drug-induced liver injury, KIBIT Cascade Eye suggests a possible association with HNF4A, which is known to cause drug-induced liver injury.”
The spiralling costs of traditional drug discovery methods mean different methods are needed. While the conventional view is that published research has yielded most of its secrets, FRONTEO’s KIBIT suggests a different picture. This new approach is timely as research and development for a new drug typically costs more than US$1billion2.
“Using our techniques, we can identify relationships not yet documented,” says Toyoshiba. “This capability sets FRONTEO apart from other NLP companies.”
References
1. Harris, Z. Word 10, 146-162 (1954).
2. Wouters, O. J. et al. JAMA 323, 844-853 (2020).
Download
Download
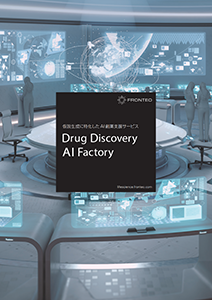
Drug Discovery Support Service
Drug Discovery AI Factory
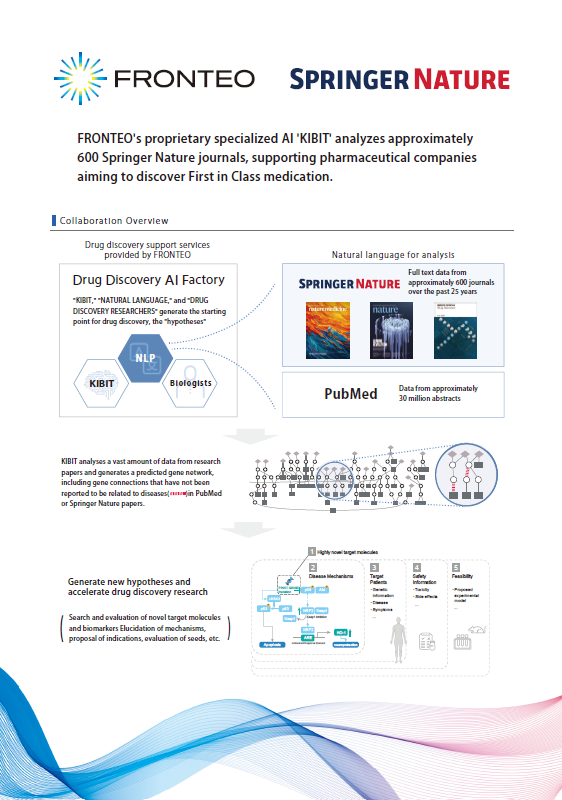
Drug Discovery Support Service