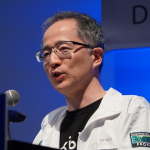
KIBIT applied to hypothesis generation AI for drug discovery, New AI drug discovery support service”Drug Discovery AI Factory”
September 26, 2023Report on FRONTEO Webinar held on 6/26/2023
"Utilizing Natural Language Processing AI to Generate Hypotheses in the Search for Novel Influencing Factors in Drug-Induced Liver Injury."
Presenter Profile: Makoto Miyamoto,
Director, Research Team, Institute for Neuro-Linguistic Science, FRONTEO Inc. Dr. of Agriculture
Graduated from Kyoto University graduate school. At Takeda Pharmaceutical Company Limited, he was engaged extensively in preclinical safety evaluation, special toxicity (phototoxicity) evaluation, elucidation of toxicity mechanisms, and safety biomarker searches from the early to late stages of new drug development.
FRONTEO is bringing innovation to drug discovery research with its "Drug Discovery AI Factory" concept, which utilizes AI from "target molecule selection”. In accordance with this "Drug Discovery AI Factory" concept, Minoru Miyamoto, Director of FRONTEO's research team, will discuss hypothesis generation in the search for factors affecting "drug-induced liver injury DILI".
Express similarity objectively by converting words into vectors (numerical values)
Makoto Miyamoto : First, I will explain about our natural language processing AI, and then introduce the Drug Discovery Best Known Methods that make full use of natural language processing AI. Then, I will explain the application of one of the methods, Vector Additive Analysis, in the field of toxicology.
An important basic concept in natural language processing is the distributional hypothesis. This is the idea that a word has meaning depending on the words around it.
For example, in the slide above, all three sentences contain the word "alcohol," but you can see that the meanings of three “alcohol” are slightly different depending on the words before and after.
That is, for a given word, in this case alcohol, we can represent the word as a vector, or number, by counting the frequency of occurrence of the surrounding words, e.g., "120 for content" and "145 for drug".
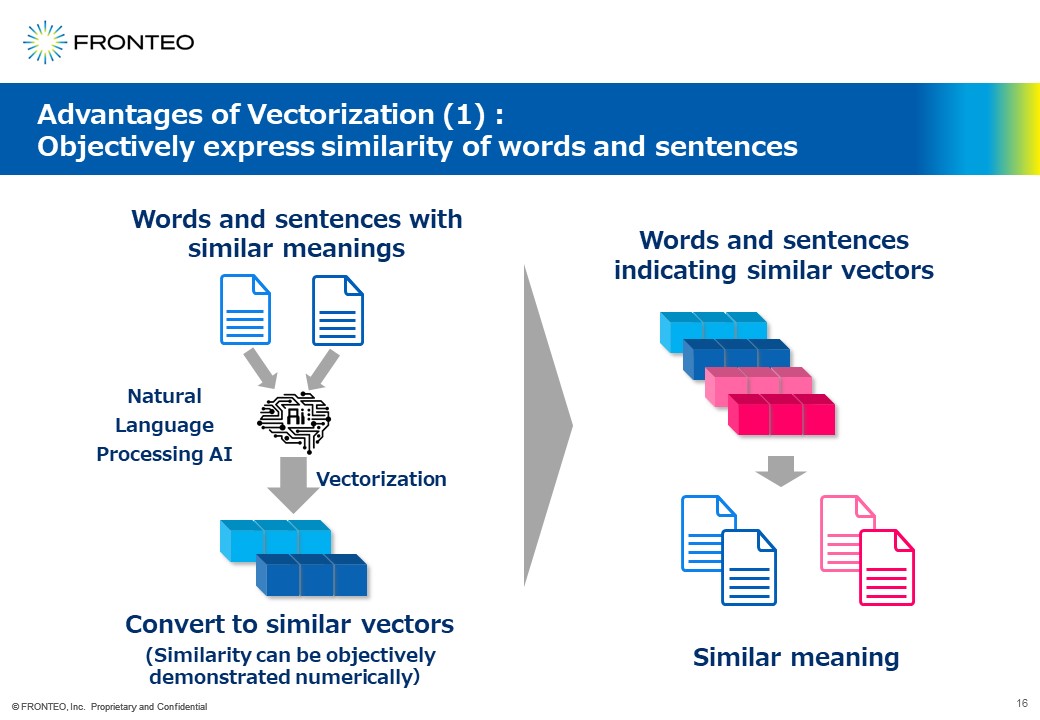
So what is the benefit of vectorization? It allows us to objectively express the similarity of words and sentences. For example, it is quite difficult to show similarity of , but when this is vectorized by natural language processing AI, the similarity can be objectively shown in numerical values.
Target molecules can be found by "addition and subtraction" with vectorization

Another advantage of vectorization is to enable to add and subtract. As a common example, if we define a " king " as a " man of authority " and subtract the concept of "man" from it, The concept of "authority” may be left. Therefore, if we add the concept of "woman" to it, it is left with the concept of "woman of authority," that is "queen.
Applying this approach to drug discovery, for example, if we want to find a drug target for ADHD, we subtract "schizophrenia" from "DRD2," which is the target for schizophrenia, and it is left with the concept of a “target”. Adding "ADHD" to it, the concept of "ADHD target" is obtained.

More importantly, new connections may be discovered. For example, look at the upper panel created from "original information" on the slide above. Here are two Francesco’s and their attributes are shown.
Interestingly, NLP AI will show different connections from the upper one. Look at the lower panel created by NLP AI. Since two Francesco’s have the same name, the NLP AI will judge that they are highly similar. Then, since Francesco (green) is an Italian male, the AI will predict that another Francesco (orange) is also an Italian male. Therefore, you may discover the possibility of new connections.
AI Engine x Drug Discovery Researchers = Drug Discovery Best Known Methods

We have developed our own AI engine, KIBIT, which is strong in natural language processing and has been patented in both Japan and the United States. KIBIT is already being used not only in AI drug discovery, but also in a wide range of life science fields.
At FRONTEO, drug discovery researchers have developed a new analysis platform called Drug Discovery Best Known Methods utilizing the AI engine KIBIT.
The network of target molecules is very important in this Best Known Methods. This network is based on a completely different concept from general ones. First, train AI with a large amounstof linguistic information. Then, use AI to predict the relationship between genes and diseases by creating a predictive model of the relationship.

If is the AI judges that there is relationship between the gene and the disease using the relationship prediction model, the AI will further build the causality prediction model. Here, if a disease is caused by gene mutations, it is called " Causality Gene”. On the other hand, if the gene expression is changed due to the disease, it is considered a " Responsive Gene ". Then, placing the causality genes upstream and responsive genes downstream, and they are connected by an interactome.
Create a comprehensive network of target genes in just 10 minutes

Such a comprehensive network can be created in just 10 minutes.
Also, as explained earlier, it is very difficult to create the network using conventional methods since the network includes new molecular (gene) connections.
Intrigulingly, networks can be created not only for "diseases " but also for "cell function," "drug," "toxicity," and so on.
Achieving gene profiling based on overlaps and/or differences between networks
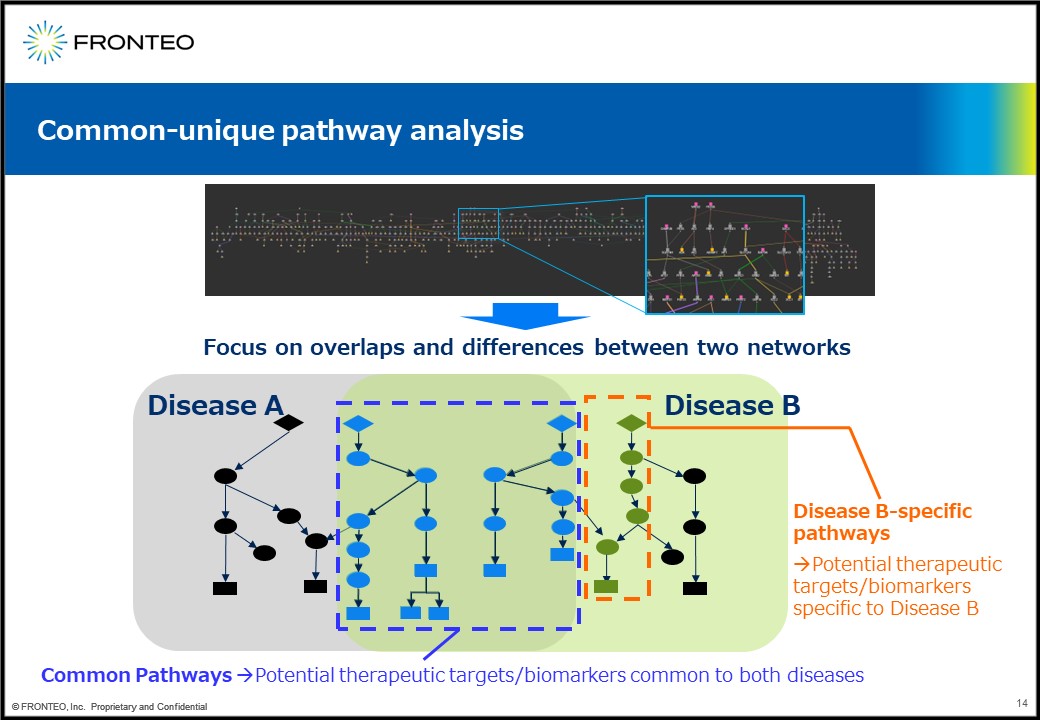
So what can we do with these networks? Our first idea is to perform common-unique pathway analysis. For example, create a network for each of two diseases, disease A and B, and then superimposing them. The blue dotted line (in the figure above) may contain a common therapeutic target and biomarker for the two diseases.
On the other hand, this orange dotted line contain a pathway specific to Disease B, so it is expected to contain targets specific to Disease B.

Next, when apromising gene is found, a method called “Virtual Experiments” can be applied for knocking out the gene in the network virtually. For example, suppose that such genes exist in the pathways in the area circled in yellow.
First, knocked out Gene A virtually. When Gene A is just replaced by Gene C without any other changes, Gene A is supeculated to have less effect on the disease.
On the other hand, when Gene B is knocked out in this way, followed by drastic changes of the entire network, Gene B can be interpreted as a key gene for the disease.
We believe that this method can be used not only for target molecule discovery, but also for safety profiling of genes of interest, i.e., on-target toxicity prediction.
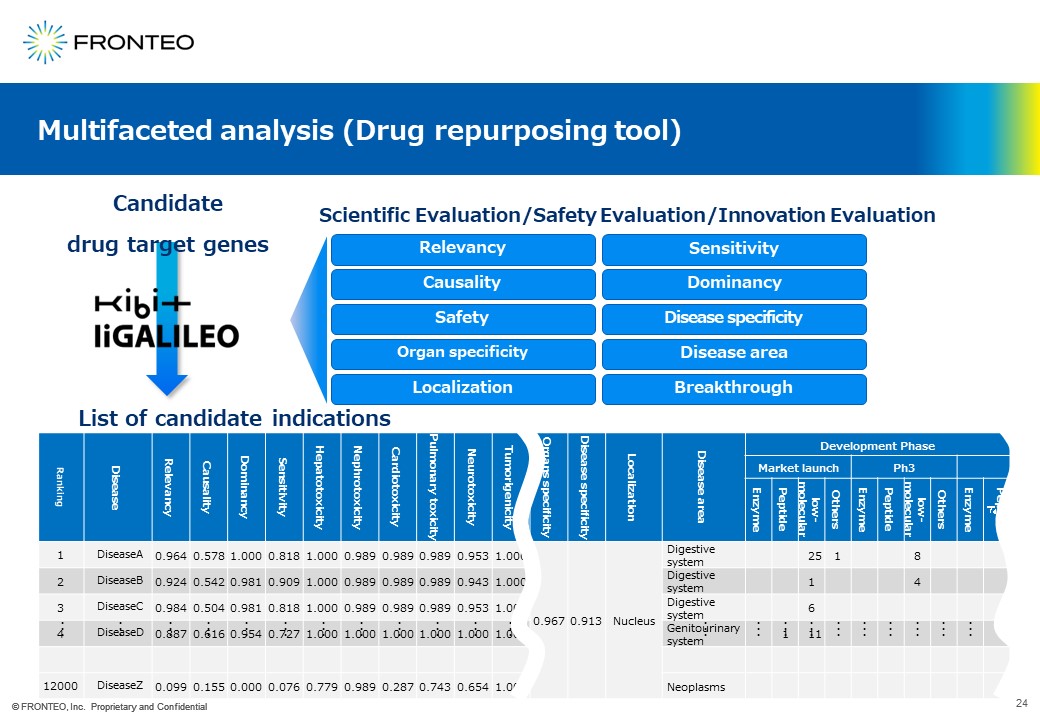
Once the gene of interest is determined, a method called multifaceted analysis can be applied. The liGALILEO can provide, a ranking of indications for the gene based on scores or information on multiple important parameters (e.g., "relevancy," "causality," "safety," and "breakthrough," etc.).
Currently, it is possible to rank approximately 12,000 diseases, including rare diseases. We believe that this multifaceted analysis is very useful for drug repositioning.
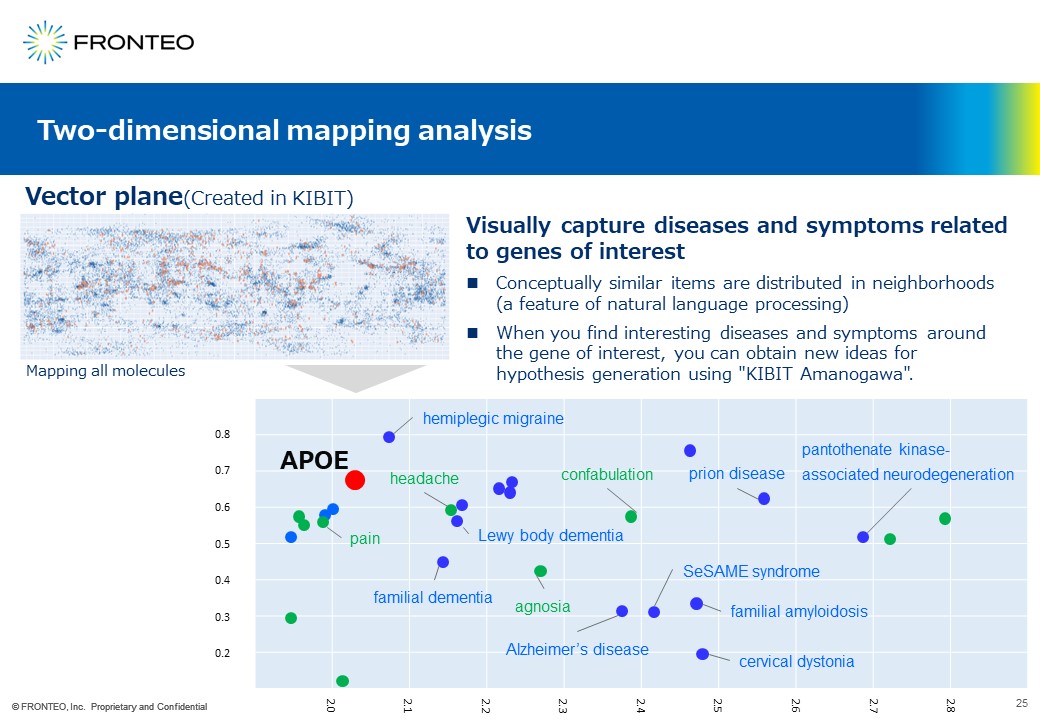
This slide represents the concept of a two-dimensional mapping analysis. This method is slightly different from the aforementioned methods. First, we vectorize various genes, diseases, and cell functions with our own AI engine, KIBIT. It is then plotted on a two-dimensional plane, a vector plane, like this.
In this way, for example, diseases and symptoms that may be conceptionally related to the gene of interest can be visually captured. This method takes advantage of the natural language processing feature that conceptually similar items are distributed proximally.
For instance, APOE is knonw to be the causative gene of Alzheimer’s disease. It is not surprising to find "familial dementia" and “Lewy body dementia “ around APOE.
On the other hand, you can find “pain” and “headache” around APOE. We believe that this is an epoch-making tool that allows us to visually grasp the relationship between molecules and diseases that we have never noticed before.
Uniting of NLP AI and drug discovery researchers enables our possibility to expand infinitely.
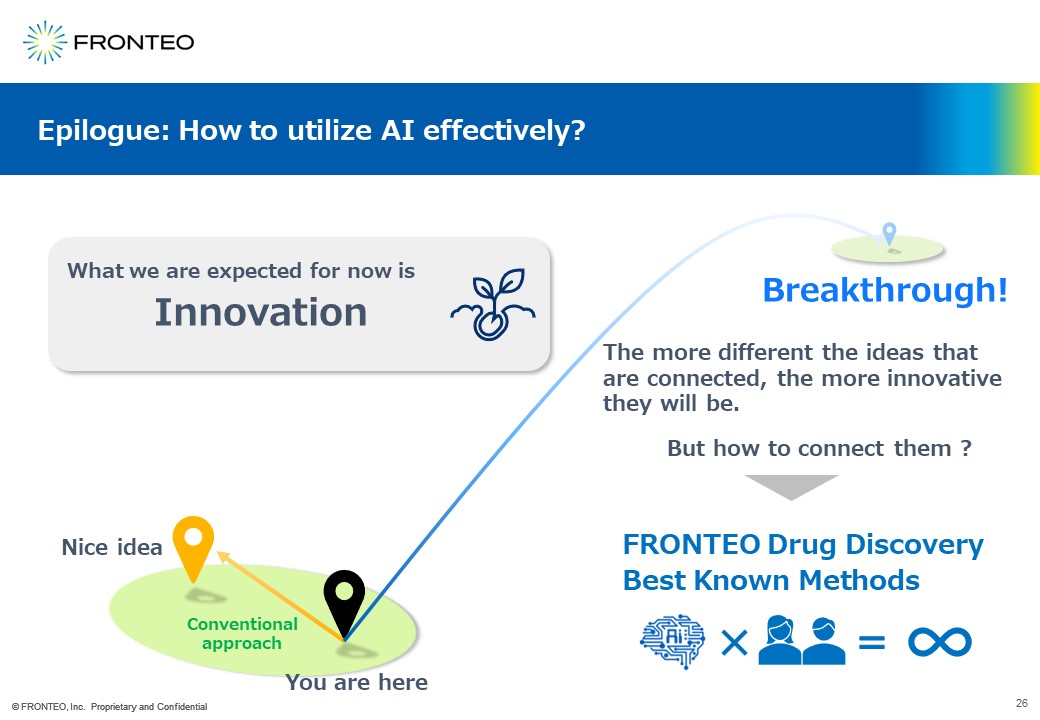
What we are expected for now is "innovation.
The more different the ideas that are connected, the more innovative they will be.However, the point is how to connect them?We believe that our Drug Discovery Best Known Methods are very effective for enabling researchers to come up with innovative ideas.
The drug discovery best known methods can make the greatest contribution to drug discovery when comprehensive and objective features of natural language AI is combined with the valuable knowledge and experience of researchers.