
“Utilizing Natural Language Processing AI to Generate Hypotheses in the Search for Novel Influencing Factors in Drug-Induced Liver Injury.”
August 23, 2023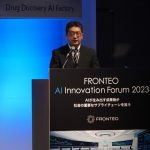
Data-driven Drug Discovery Initiatives at Mitsubishi Tanabe Pharma Corporation”
November 16, 2023Report on FRONTEO Webinar held on 6/26/2023
KIBIT applied to hypothesis generation AI for drug discovery, New AI drug discovery support service"Drug Discovery AI Factory"
Presenter Profile : Hiroyoshi Toyoshiba,
Executive Officer, CTO, FRONTEO Inc. Doctor of Science
Since 2017, he has been engaged in life science AI development at FRONTEO. He developed an AI algorithm specialized for the life science domain. Taking advantage of the feature of text vectorization, he has developed various businesses based on this algorithm, such as thesis search, drug discovery support, dementia diagnosis support, fall prediction, etc. Life Science AI CTO since 2019. Appointed as Executive Officer in 2021. Further promote the social implementation of AI.
FRONTEO is bringing innovation to drug discovery research with its "Drug Discovery AI Factory" concept, which utilizes AI from "target molecule selection”. Dr. Hiroyoshi Toyoshiba, Ph.D., the person in charge of the "Drug Discovery AI Factory" concept, talked about the world of drug discovery he is aiming for and FRONTEO's current approach to drug discovery.
“First in class” is the mainstream of drug discovery today
Hiroyoshi Toyoshiba: I will focus on our drug discovery support service "Drug Discovery AI Factory". First, I believe there are two important factors to win in the drug discovery field.
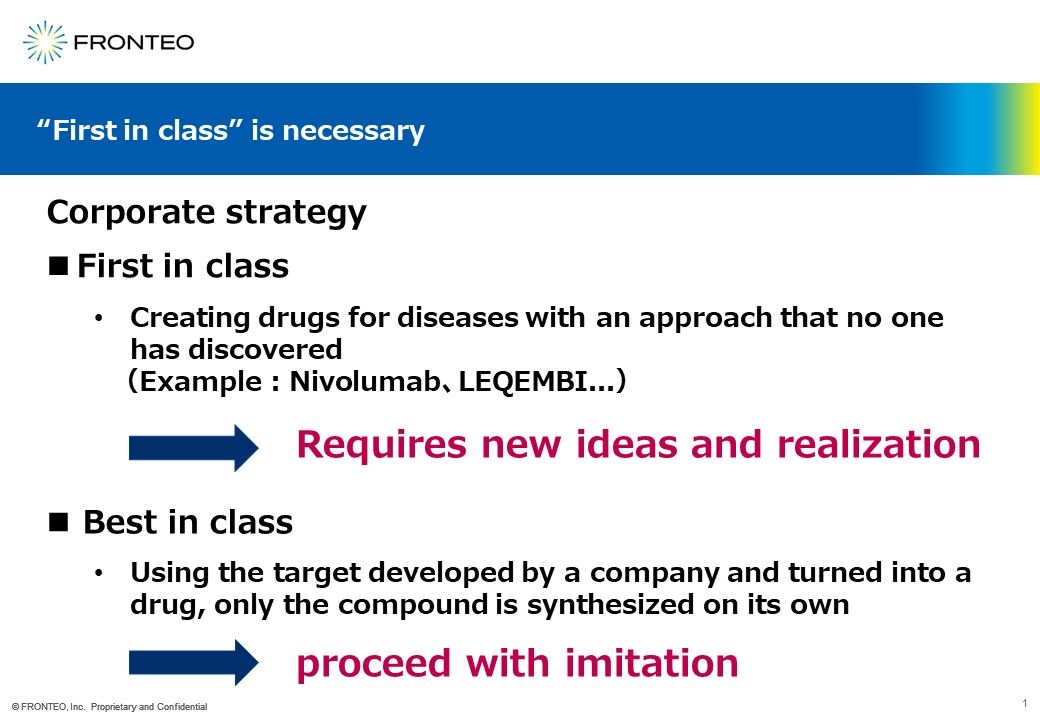
The first important element is “First in class” (creating drugs using unconventional approaches). It is becoming increasingly difficult to bring a drug to market unless it is first in class as a corporate strategy.
But if you want to be “First in class”, you need new ideas and the ability to make them happen.
Until a decade ago, most Japanese pharmaceutical companies developed drugs based on the “Best in class” approach (searching for a candidate compound with higher efficacy and lower toxicity based on the structure of a Fist in class compound).
“Best in class” can be done to some extent by "copying" existing products, but the current mainstream, "first in class," requires new ideas.

New ideas are not something you can just come up with. It is a very difficult task to propose a target that has little or no actual relevance to the disease.
In fact, when I try to find them, I can only recall one or two molecules that I have seen, and the "coming up with new ideas" required for “First in class” is extremely difficult.
"At least one per year" - number of projects to produce new drug

Another important factor is "number". The number 24.3 here refers to the number of projects needed to produce at least one new drug in a year. Since there are about 50 weeks in a year, dividing by 24.3 means two projects per month or one every two weeks. We need new targets at this rate.
So what does it take to create this 24.3? It is also states that "AI-based selection of drug targets" based on human data will be necessary, according to a document published by the Ministry of Health, Labour and Welfare (MHLW).

The yellow mark indicates areas where AI is used to a large extent. AI is used to some extent in virtual screening, compound synthesis, optimization.
As for the clinical trial section on the far right, it is a green smiley face symbol, which means that AI is being used quite well.
However, the most important part of the drug discovery process, which has been discussed earlier, is on the left. The reality is that AI cannot be used very well in this target selection part.
FRONTEO would like to advance its business by utilizing AI, especially in the initial idea conception and target molecule search and selection phases.
The key to this phase is the selection of novel target molecules and its hypothesis generation. When researchers consider a target molecule, they tend to base their thinking on the information in the literature they have read, but KIBIT enables comprehensive and unbiased analysis of the 30 million publications in PubMed*.
Furthermore, even if a gene is not directly mentioned in a paper, if a gene with a similar function or position to the gene associated with a disease is mentioned in a different paper, KIBIT will predict the association with the disease and connect it to the disease just as a researcher would guess, making it possible to find highly novel target molecules that could not be found using existing approaches such as gene expression analysis or GWAS.
*Biomedical domain article database operated by the National Biological Sciences Information Center, U.S. National Library of Medicine.

I have handled a variety of data in my previous jobs, and most of the data that comes out of this place are basically "lists". For example, we get lists of genes, compounds, and so on.
However, even with a list of highly novel target molecules, it is very difficult to move forward in the drug discovery process without a hypothesis. Estimating a hypothetical disease mechanism generally requires researchers to read a vast number of papers. At the Drug Discovery AI Factory, which has strengths in hypothesis generation, our drug discovery researchers with expertise in AI and drug discovery utilize KIBIT to generate disease mechanism hypotheses in a short time.
In order to continue to achieve this number of 24.3, we believe it is important to continuously generate novel and testable hypotheses. This is where we would like to propose a different approach using AI.

One of the characteristics of our AI is that it is very good at natural language processing. We use a technique called distributed representation as the basis of our AI.
The underlying theory follows the simple rule that a word is characterized by the words around it. We are not asking an AI to recognize the word "alcohol" as it is here.
The AI recognizes the word alcohol based on the type and frequency of the words that appear around it. So in the case of our engine, the reason it determines that the two words have similar meanings is because of the type of words that appear around it and their frequency.
According to these rules, our AI evaluates the proximity of sentences and words.
“What Feels Like Noise” Leads to Innovation
Some of our clients have told us that "the noise is coming up" using our AI. However, basically, our AI does not generate noise due to its algorithm.
We perceive noise because the meanings of the words we are assuming are very limited. In other words, we do not have a picture of what the word means beyond what we have read in the literature so far.

This unbiased, so-called meaning making according to certain rules becomes very important. It is often said that innovation is brought by "intentional coincidence" and "fusion of different fields," and I believe that these unbiased results produced by our AI, the "things that feel are noise" I mentioned earlier, are themselves I think "intentional coincidences" and the beginning of new connections (fusion of different fields).
We intend to use our AI in this way as a tool that will approach us in a new way, or rather, in a way that we are not easily aware of.
So why do we need drug discovery researchers there?
Combining AI and researchers to generate hypotheses
There is a famous story about Edison who, when he invented the light bulb, was very worried about what substance to use for the filament. In the end, he happened to see a bamboo fan on his desk and came up with the idea of using bamboo for the filament.
I think it would be difficult to connect the "new connections" that our AI will bring out with our past knowledge and past drug discovery research, unless you have specialized in this field, just like this bamboo and filament.
Therefore, we would like to "generate new hypotheses" in such a way that our AI and researchers are merged together.
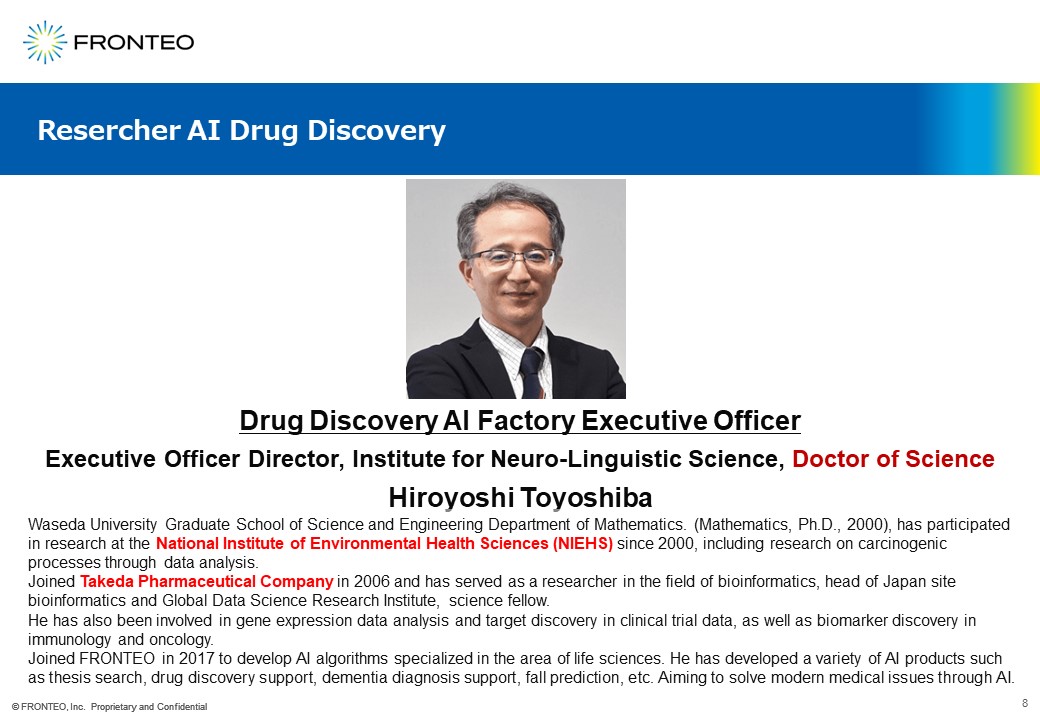
I have been involved in drug discovery research for many years in my previous position, and other researchers here have also been involved in drug discovery research for many years in pharmaceutical companies.

We hope to combine these researchers with AI to generate new hypotheses.
AI x Best Known Methods Created by Drug Discovery Researchers
In fact, our in-house developed AI and our drug discovery researchers have developed five methods called Best Known Methods.
This method was not developed simply by a researcher who has been working on AI for a long time and thought it would be useful for drug discovery research, but was created by a person who has actually conducted drug discovery research from the viewpoint of how our AI can be used for drug discovery research, so we think it’s very useful method.
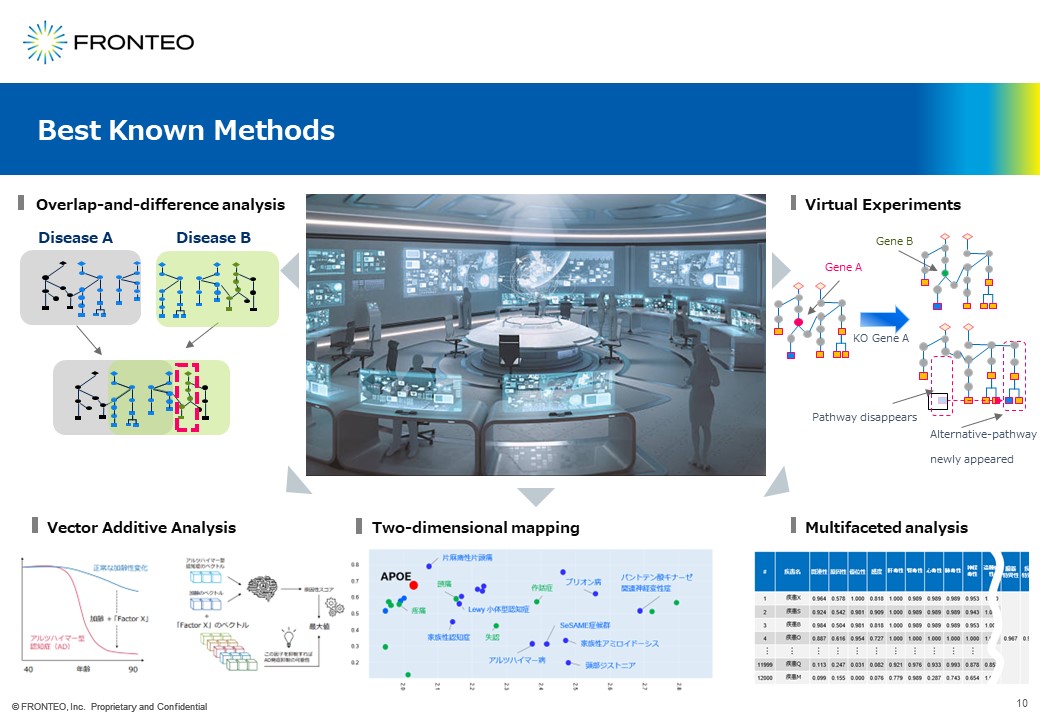
This Virtual Experiments, which is up here on the far right, represents the connections between diseases and molecules in the form of a network. Knock out one molecule in the network then we analyze the pathways that emerge afterwards. For example, if this pathway is gone, we select a new target molecule by looking at what would emerge in its place.

In the case of our AI, if you enter the name of the disease, in about 10 minutes, it will show you a target molecular network diagram of about 700 targets like this.
In this process, the AI will also predict and make connections between genes that are not directly described in the paper. Our researchers will raise which target molecules are most interesting for the part of the network that they have focused on, using an analysis recipe that combines the five methods of the Best Known Methods.
Let’s say this blue area (blue circle) corresponds to the molecule. Our drug discovery researchers will look at the various functions and properties of the molecule and will ultimately lead to a hypothesis. I think this is a characteristic of our AI drug discovery.
Five Target Molecules Discovered in Just Two Months

Finally, I would like to share with you some of our accomplishments to date. To date, we have worked on more than 20 projects on a contract basis.
As you can see in the middle of this page, the contents include target search, indication search, drug combination, and biomarker search.
We have heard that more than 30 of our proposed targets have already gone to Vitro(Cell Experiments) and shown efficacy, and more than 5 have gone to Vivo(Animal Experiments). The output is what comes out of the cross-fertilization of our AI and researchers.

Here are the latest results. As I mentioned “First in class” at the beginning of this report, we have nominated about 100 target molecules for analysis using this network in about two months. This project has been very challenging, and we have identified an additional 20 disease-relevant target molecules that have not yet been reported among this 100.
We were told that the 20 proposed target molecules were actually studied in vitro, five showed phenotypes, and one progressed to vivo.
We will propose novel and high quality target molecules by looking at the pathways. We will propose not only the pathways that are connected up and down, but we will also recognize whether or not it is a different mechanism on our part and where the pathways has been selected.
I also believe that the selection of five previously unreported target molecules in a very short period of time (two months) was the result of an unprecedented approach to analysis.

Current drug discovery requires "first in class". For this, we were able to propose molecules that are novel and that span multiple criteria for adoption. We were able to propose 20 target molecules that are highly novel because their connection to the disease is not directly described in the paper.
Next is the quantitative portion of the 24.3 target molecules needed to bring to market. Here, too, five out of 20 showed phenotypes in a short period of two months.
Since there are five in two months, that is 2.5 per month. Since our initial target of 24.3 units was more than 2 units per month, we believe we have achieved our goal of supporting drug discovery in terms of quality and quantity.