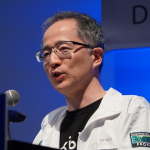
KIBIT applied to hypothesis generation AI for drug discovery, New AI drug discovery support service”Drug Discovery AI Factory”
September 26, 2023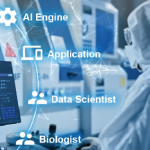
[Basic Information] What is AI Drug Discovery?
November 20, 2023Report on FRONTEO Webinar held on 7/19/2023
Data-driven Drug Discovery Initiatives at Mitsubishi Tanabe Pharma Corporation
Presenter Profile : Ryuta Saito, Ph.D.
Principal Research Scientist
Discovery Technology Laboratories
Sohyaku. Innovative Research Division
Mitsubishi Tanabe Pharma Corporation
Ryuta Saito of Mitsubishi Tanabe Pharma Corporation presented a session on the company's drug repositioning work and drug target discovery conducted in collaboration with FRONTEO at the FRONTEO AI Innovation Forum 2023 on July 19, 2023. Dr. Saito, who has been involved in the field of drug discovery for many years, will explain how AI can solve problems in drug discovery and where the problems lie in the future, based on the drug target search conducted jointly by Mitsubishi Tanabe Pharma Corporation and FRONTEO.
Moving Away from High-Risk, High-Return Drug Discovery
Ryuta Saito: I have been promoting drug discovery at Mitsubishi Tanabe for the past 20 years, focusing on bioinformatics. I am in charge of not only bioinformatics and omics analysis, but also digital transformation of R&D.
In today's presentation, I will first discuss our company's DX efforts. After that, I will introduce our data-driven drug repositioning efforts, and lastly, I will talk about a case study in which we conducted a target search together with FRONTEO.
First, let's look at the evolution of informatics. 20 years ago, the human genome was read, and since then, technology has advanced and computer power has increased rapidly, so that we are now dealing with very large amounts of data. Not only large amounts of data, but also various types of data are being handled.
In line with this, informatics has been required to play a variety of roles, and over the past 20 years, we have been considering the incorporation of analysis techniques that we believe are necessary on an ad hoc basis.
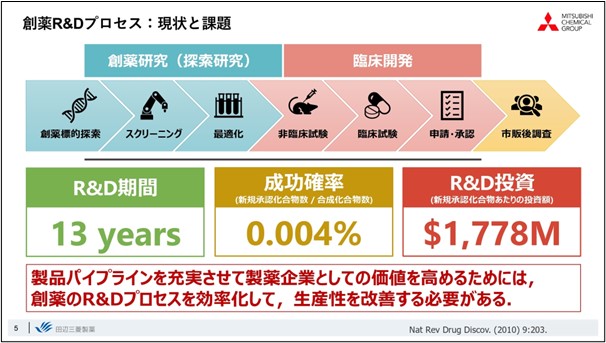
Drug discovery is characterized by a high-risk, high-return structure, with extremely long R&D periods and almost all of them failures. So productivity is very poor. In order to enhance the pharmaceutical pipeline and increase corporate value, this productivity must be improved.
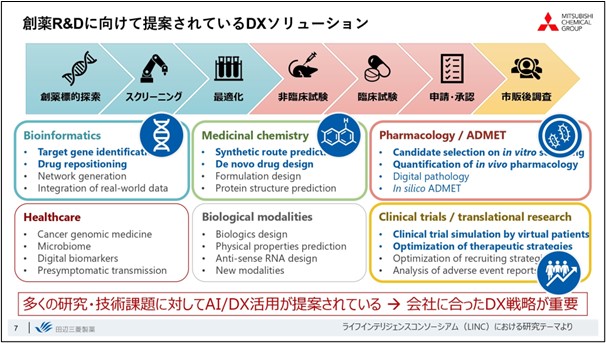
To achieve this, it is necessary to extract new value from the large amount of data that has been collected and acquired thus far, and to increase productivity by efficiently promoting drug discovery R&D.
Drug discovery is an "integrated science" in which success or failure is determined by the aggregation of a great number of technologies. Since there are so many situations in which AI can be used, it is important to properly establish the technologies that match the company's strategy. We have selected about four of these technologies that fit our overall strategy and are promoting them as key technologies.
As the Pharma section of the Mitsubishi Chemical Group, we have set a vision of becoming a "healthcare company that delivers optimal medical care to each and every individual. In order to realize this vision, we have set two major goals for R&D.
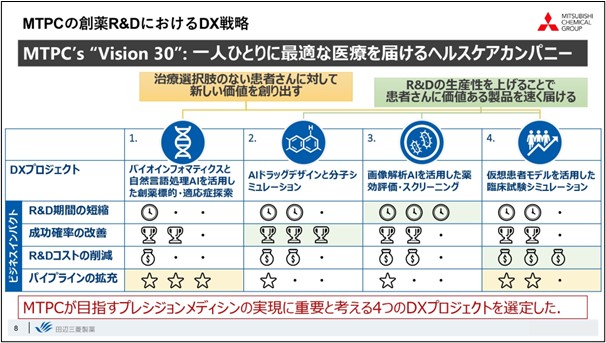
The first goal is "to create new value for patients who have no treatment options. And the second goal is "to deliver valuable products to patients faster by increasing R&D productivity” as we believe it is important to quickly deliver this new value to patients who need it.
The main direction of our DX strategy is to achieve both of these goals. Regarding the four key technologies I mentioned earlier, the first is " bioinformatics, and drug target/indication discovery utilizing natural language processing" which is being researched primarily for the purpose of creating new value. The second is "AI drug design molecular simulation. The third is "drug efficacy evaluation and screening utilizing image analysis AI," and the fourth is "clinical trial simulation using virtual patient models. These three key technologies will mainly help improve the efficiency of drug discovery research and increase the probability of success. Not only use these four key technologies to improve the efficiency of drug discovery research, but also promote the creation of "new value" by applying the improved efficiency to the next idea.
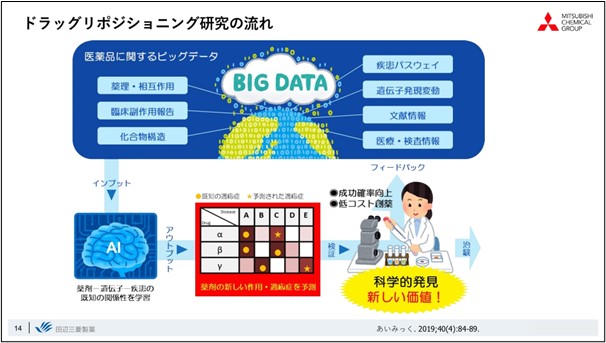
Let me talk about "drug repositioning" as the first example of data-driven drug discovery. I have montioned earlierthat the Drug discovery is characterized by a high-risk, high-return structure, the Drug repositioning is the process of finding new indications for compounds that are already on the market or in the process of development and providing new value.
Compared to conventional drug discovery methods, this approach is attracting attention because it enables drug discovery with reduced risk due to its ability to determine safety and other factors, and it is estimated that about one-tenth on the cost. However, until now drug repositioning has been based on the knowledge and ideas of doctors and researchers, and we are considering the possibility of using large-scale data and AI technology to accelerate this process.
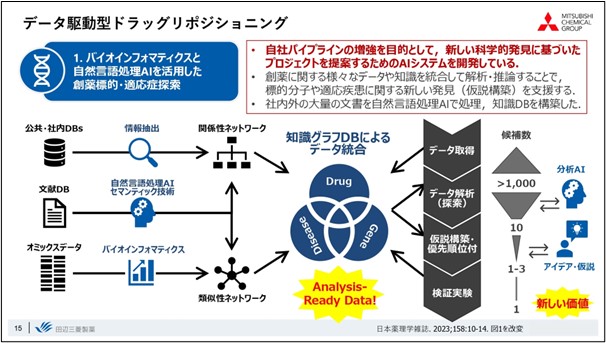
The overall flow of drug repositioning research is to predict new relationships AI technology and other methods,based on big data related to drugs, such as drug efficacy and clinical trial data, compound information, disease and compound-related pathway information, gene expression, and literature, utilizing. By experimentally verifying this data, we are trying to create scientific discoveries, or new value.
We use natural language processing primarily when dealing with literature data, and we are creating Knowledge Graph databases, such as those that relate diseases to drugs and genes, based on databases already incorporated in-house and the results of bioinformatics analyses.
Using this Knowledge Graph database, when a researcher wants to find a new indication for a drug, an initial search will yield approximately 1000 candidate indications. Utilizing analytical AI techniques to further narrow down the list, and combining it with the researcher's ideas and the results of overall data analysis, the number is carefully selected to the point where it is possible to conduct an experiment.

Regarding the approach to drug repositioning using the Knowledge Graph, I would like to present one symbolic case study related to Covid-19, although it is a case study of another company. This case study is from a company called Benevolent AI. Using machine learning, the relationship between genes and symptoms related to the disease was described in a Knowledge Graph, and based on the mechanism of infection and disease progression of Covid-19, the genes involved in severe disease and the drugs that act on them were analyzed.
The drug “Baricitinib” is coming up in this analysis. This paper came out in February 2020. Then Eli Lilly, the company that has this “Baricitinib”, had a successful clinical trial that showed that it significantly reduced mortality in hospitalized patients, and the FDA approved it in November 2020.
I mentioned earlier that it takes more than 10 years for the research and development of a drug, but in this case, it took less than a year from the publication of the paper to the actual approval by the FDA, which I think was a very significant event.
Of course, it was a global pandemic, and it happened because of the push of social demands, but it was also an example of how the value created by data drive can quickly survive development trials, and I for one was encouraged by it.
Natural Language Processing AI Accelerates Drug Repositioning

In fact, let me explain how we are conducting repositioning research in our company. As one of the base technologies, we have been studying Word2vec for about 6 years, a concept similar to that of FRONTEO's AI engine "KIBIT". This is a technology based on a neural network that learns the relationship between the word and its surrounding words, converts it into a vector, and is able to perform arithmetic operations related to the word.

This is a slide that illustrates an example that is often used in explanations. I find it interesting that the potential vectors connecting each word to each other hide their intrinsic meanings.

When we do this Word2vec analysis in the drug discovery field, Drugs is mapped to the position of the addition of Disease and Genes, respectively. Using these properties, there is a possibility to derive mathematically by arithmetic operations, including those we are not yet aware of.
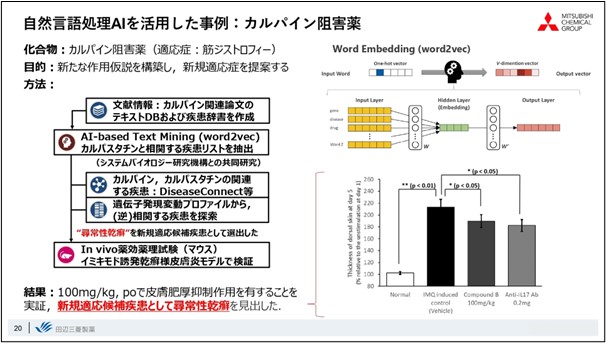
Here is one case study of the use of Word2vec. This is a case study about a drug discovery concept called calpain inhibitors, a drug target that has been well known for a long time and was probably done by every pharmaceutical company about 20 years ago.
We examined the possibility of finding entirely new indications for Word2vec using calpain inhibitors as the subject matter. First, we created a list of diseases that correlate with calpastatin, an endogenous inhibitor of calpain, in the word space, and by overlaying gene expression data and contributions in disease-related pathways with database analysis, a previously unnoticed skin disease called psoriasis vulgaris emerged as a candidate indication. By overlaying the database analysis with gene expression data and contributions in disease-related pathways, a previously unnoticed skin disease, psoriasis vulgaris, emerged as a candidate indication.
We actually tested the pharmacological effects predicted by Word2vec in a mouse model of imiquimod-induced psoriasis-like dermatitis. As a result, we found that the calpain inhibitor exhibited a similar level of efficacy as the previously marketed anti-IL-17 antibody. The results show that we have not only predicted a new indication, but have also properly validated it.

Here is another example of drug repositioning. This one does not use natural language processing techniques in the discovery phase, but rather uses machine learning techniques to discover new relationships between genes, compounds, and their indications for PPAR agonists, another very well-known class of drug compounds. If we can find a new target molecule for a compound, we can build a concept based on the new discovery, which is quite advantageous when considering indications. This is the result of machine learning to predict what the new target molecule will be.
This is a joint research project with Yamanishi at Nagoya University(at the time Kyushu University). We obtained 23 new candidate target molecules through machine learning. Among them, we selected 18 compounds that we could actually conduct experiments on, and conducted experiments to see if the compounds actually bind to the predicted target molecules.
As a result, we found that 9 out of 18 molecules actually bind. One of them is PPARG, which has been known from the beginning, but a new molecule, MAOB, was found to be stronger than PPARG.

Once a new target molecule, or pharmacological action, is identified, the next question is how to consider indications. This is where we used natural language processing. Here, we focused not on Word2vec or anything like that, but on phenotypic words that describe genes and disease symptoms, and analyzed the words that co-occur in sentences for each disease to create a profile that describes the disease.
The following is the result of mapping diseases with similar profiles to insulin resistance, originally known as PPAR, and Parkinson's disease, well known as MAOB. In this way, it has become clear that some neurodegenerative diseases, cerebral infarction, and other diseases that we had not paid attention to before are mapped as similar diseases.
In the two case studies presented today, we have shown natural language processing techniques that are useful in the exploratory phase of drug repositioning research, and natural language processing techniques that are useful in the validative phase and in prioritization, respectively. As demonstrated in these cases, we believe that natural language processing techniques can work very powerfully in narrowing down candidate indications.
Collaboration with FRONTEO for novel target discovery

Finally, as an example of collaboration with FRONTEO, I would like to introduce a target search case study, in which FRONTEO's natural language processing AI technology is used to vectorize a vast amount of textual information from medical articles, and based on the vectorized results, together we create a molecular network or disease map for that disease. We are trying to use the disease maps generated in this way to search for targets of the diseases we have focused on.
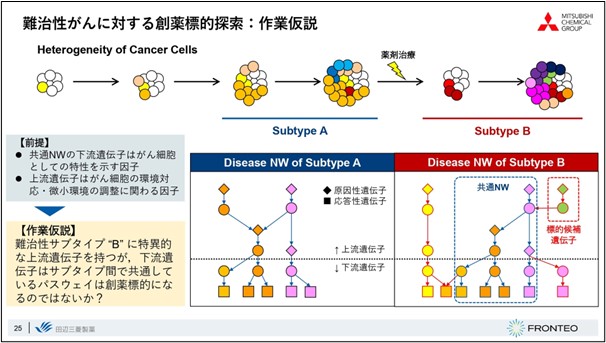
When the diversity of the disease itself is high, such as in the case of cancer, it can be assumed that the initial stage, for which treatment methods are available, is completely different from the refractory stage, for which symptoms do not improve even after therapeutic intervention. The refractory stage, in particular, consists of a very complex network.
FRONTEO and I discussed how to find drug targets from such a complex network, and we analyzed with the working hypothesis that "upstream genes specific only for refractory subtypes with common pathways among subtypes are one of the important causes of refractory subtypes. We analyzed the data with the working hypothesis that When we create a disease map for each disease subtype and look at the overlapping differences, we can say that the pathways that come in common are quite important for the disease state. We fixed the pathways that were downstream of the pathways as being likely to affect the phenotypes important to the condition, and searched upstream of the pathways for causative genes that are characteristic of the refractory subtype B alone. We thought that if a new pathway emerged only for the refractory subtype, and a signal was coming from there, this could be one of the candidates for a drug discovery target.
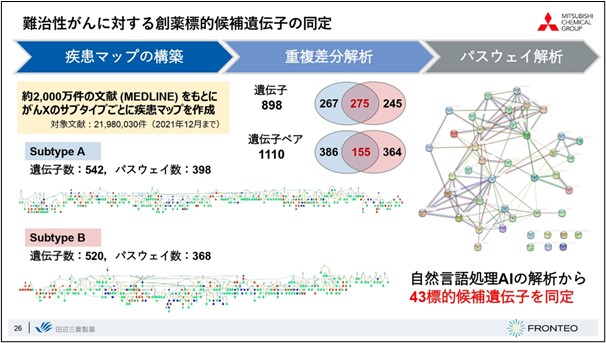
Here is an actual example. Disease maps were created for each subtype, and a differential duplication analysis revealed 275 duplicated genes and 155 duplicated gene pairs. By identifying common pathways from these duplicated gene pairs and taking the genes that are characteristic of subtype B from the upstream factors, 43 genes were identified.
We believe that the network analysis of these 43 genes has revealed a strong relationship between each of them, and that we may be able to obtain information that may form the core of some pathological condition.

To prioritize these 43 genes, we conducted Virtual Experiments, which are virtual gene knockout experiments on a network to see how the original network changes when a gene is excluded, and to determine its impact on the network. We then scored the impact of the changes, and based on the changed patterns, we developed new hypotheses. We narrowed down the 43 genes mentioned above to 12 genes and conducted Virtual Experiments analysis, which revealed commonalities in the affected pathways. By focusing on the affected pathways, we have been able to construct a hypothesis for the selection of target molecules.
We believe that we were able to extract novel candidate target genes by utilizing FRONTEO's natural language processing technology. We have not yet fully verified the results of this study, so we are looking forward to the future.
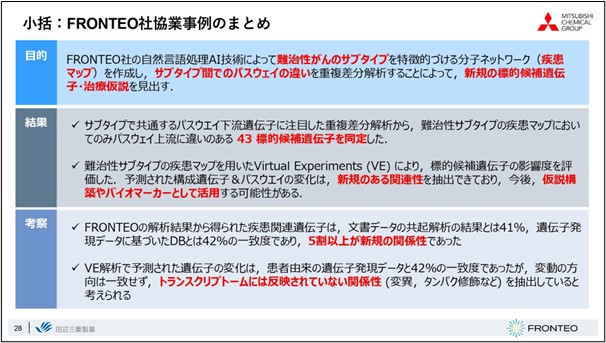
Here is a summary of what we did with FRONTEO. This is a very useful technique in terms of getting new relationships. In particular, as noted in the discussion, about 50% of the relationships that came up were new relationships. Moreover, Virtual Experiments has proven to be able to extract candidate molecules differently from bioinformatics analyses, such as transcriptomes, and we were able to demonstrate the advantages of using word space. Although it is still necessary to devise a method to increase the validity of the extracted new candidate target molecules, we believe it is a useful tool in the search for new candidate target molecules.
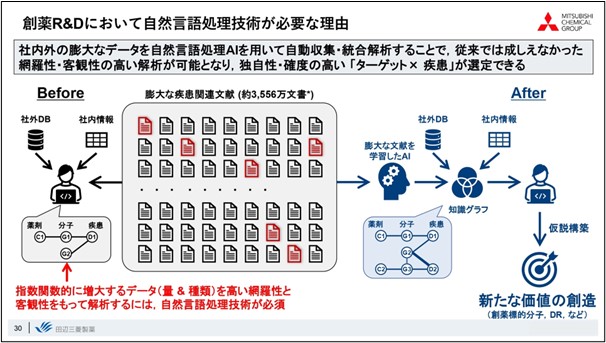
In closing, I would like to summarize. The amount of document-related information is exploding, and there is a limit to what researchers can do with the literature base they have until now. In order to systematically analyze this enormous amount of information and link it to highly original ideas, natural language processing AI technology, as introduced in this presentation, is indispensable, and I believe it is one of the most important tools for creating new value.
There are various technologies when it comes to natural language processing AI, and it is important to use each one according to the task at hand. I also feel that the current technological challenge is whether researchers can properly imagine the information hidden in the latent expression from the analysis results utilizing AI and link it to new discoveries. Since this is a field where technological progress is rapid, I hope that future technological developments will overcome these challenges and accelerate efforts toward data-driven drug discovery.